Optical seminar | 18 December 2020
Online
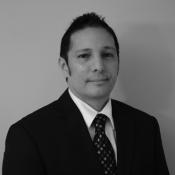
From the initial realization of negative refractive index metamaterials, research in artificial electromagnetic materials (AES) has flourished, spanning from cloaking and perfect absorption to non-reciprocity and quantum optics. Underpinning this revolution was the well-timed development of nanofabrication, and maturation of computational electromagnetic (CEM) software. Often the goal of designing and fabricating an artificial electromagnetic material (AEM) is to specify the scattering and surface or volume propagation of free-space or guided electromagnetic waves. As such, CEM software potentially allows for an efficient design loop, where modification of the AEM geometry is varied until a desired output (scattering) is obtained. For some cases, a simple geometrical parameter sweep is sufficient to achieve the desired goal. However, for more challenging outputs, it may be necessary to utilize modern optimization techniques – many of which are built-in to commercial CEM software.
The prediction and design of the scattering properties of artificial electromagnetic materials has traditionally been guided by theory, computational methods, and optimization techniques. Rapid advance in the field of deep learning has spawned significant interest in developing data-driven methods for artificial electromagnetic materials. In a data-driven approach, the large number of parameters that constitute a neural network, are used to learn a forward mapping from an input vector -- typically the geometry of the AES -- and are mapped to an output vector, which may be frequency dependent scattering. A large dataset may consist of geometry/spectra pairs, and is used to minimize the error between ground truth (numerical simulation) and the output of the neural network. I present results on deep learning developed to predict the electromagnetic response of super unit cell all-dielectric metasurfaces operating at terahertz frequencies. I also demonstrate an inverse model that yields specific geometrical parameters needed for a desired spectral response. The developed inverse method is not restricted to the case presented, but may be applied to many other systems including photonic band gap, and plasmonic structured materials.