Theoretical seminar | 20 October 2021
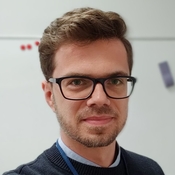
Recent years have witnessed remarkable developments in big data, artificial intelligence and neural networks. Machine learning has found wide applications in both research and the industry. This comes at the cost of high levels of energy consumption that are necessary to process large amounts of data. It is expected that over 20% of global electricity use by 2030 will be used for information processing. The performance of complementary metal-oxide semiconductors (CMOS) no longer follows Moore's law. In result, much research has been aimed at finding an alternative platform for information processing, characterized by high performance and energy efficiency.
In this talk I will review recent progress in machine learning with photons. Photonic information processing benefits from high speed, parallelization, low communication losses, and high bandwidth. Fully functional photonic neurons, including spiking neurons, as well as neural networks, have been already realized in laboratories. Several networks achieved high performance in challenging machine learning tasks, such as image and video recognition.
We recently demonstrated hardware neural network systems where strong optical nonlinearity results solely from interactions of exciton-polaritons, quantum superpositions of light and matter [1,2,3].
Such superpositions, in the form of mixed quasiparticles of photons and excitons, are characterized by excellent photon-mediated transport properties and strong exciton-mediated interactions.
These semiconductor microcavity systems can be used to construct fully all-optical neural networks characterized by extremely high energy efficiency [4]. We show why using polaritonics in place of standard nonlinear optical phenomena, is the key to achieving such a performance.
[1] A. Opala, S. Ghosh, T. C. Liew, and M. Matuszewski, Physical Review Applied 11 , 064029 (2019)
[2] D. Ballarini, A. Gianfrate, R. Panico, A. Opala, S. Ghosh, L. Dominici, V. Ardizzone, M. De Giorgi, G. Lerario, G. Gigli, Timothy C. H. Liew, Michal Matuszewski, and Daniele Sanvitto, Nano Letters 20, 3506 (2020)
[3] R. Mirek, A. Opala, P. Comaron, M. Furman, M. Król, K. Tyszka, B. Seredynski, D. Ballarini, D. Sanvitto, Timothy C. H. Liew, Wojciech Pacuski, Jan Suffczyński, Jacek Szczytko, Michał Matuszewski, and Barbara Piętka, Nano Letters (2021)
[4] M. Matuszewski, A. Opala, R. Mirek, M. Furman, M. Król, K. Tyszka, T.C.H. Liew, D. Ballarini, D. Sanvitto, J. Szczytko, B. Piętka, arXiv:2108.12648.